Resource Hub
Resources
Read whitepapers, fact-sheets and reports to expand your knowledge and stay on top of your eDiscovery game.
Blogs
Blogs
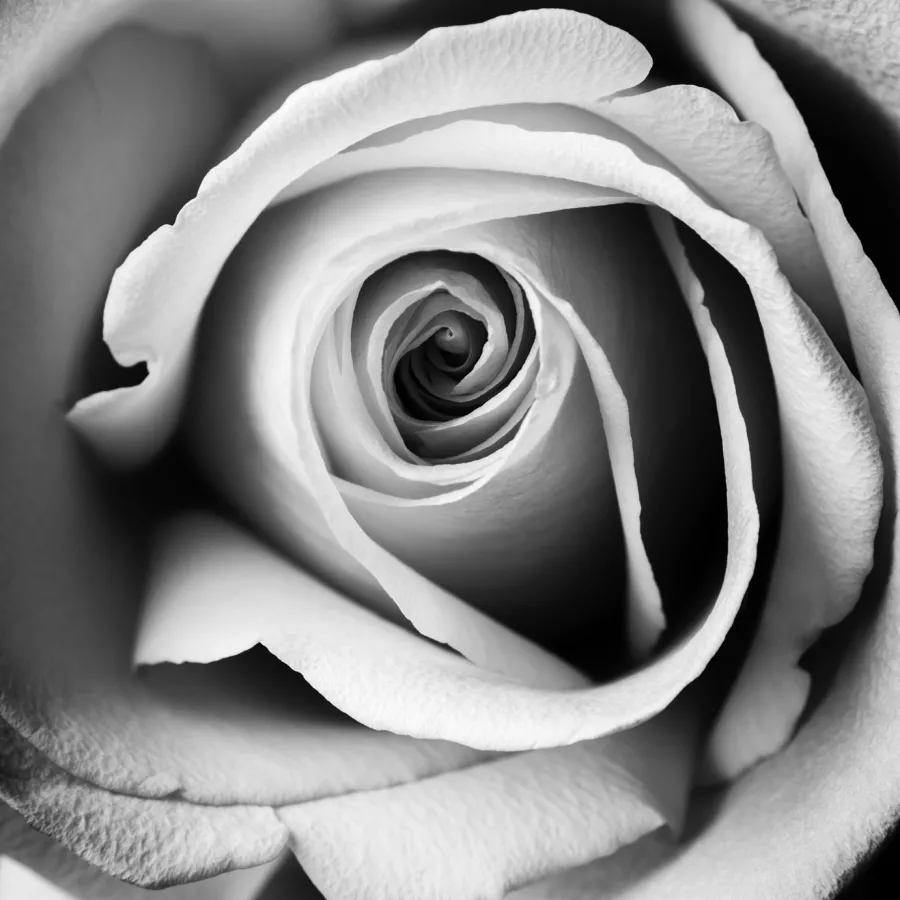
Xplore_AI: What’s in a name? Be specific!
Read more ›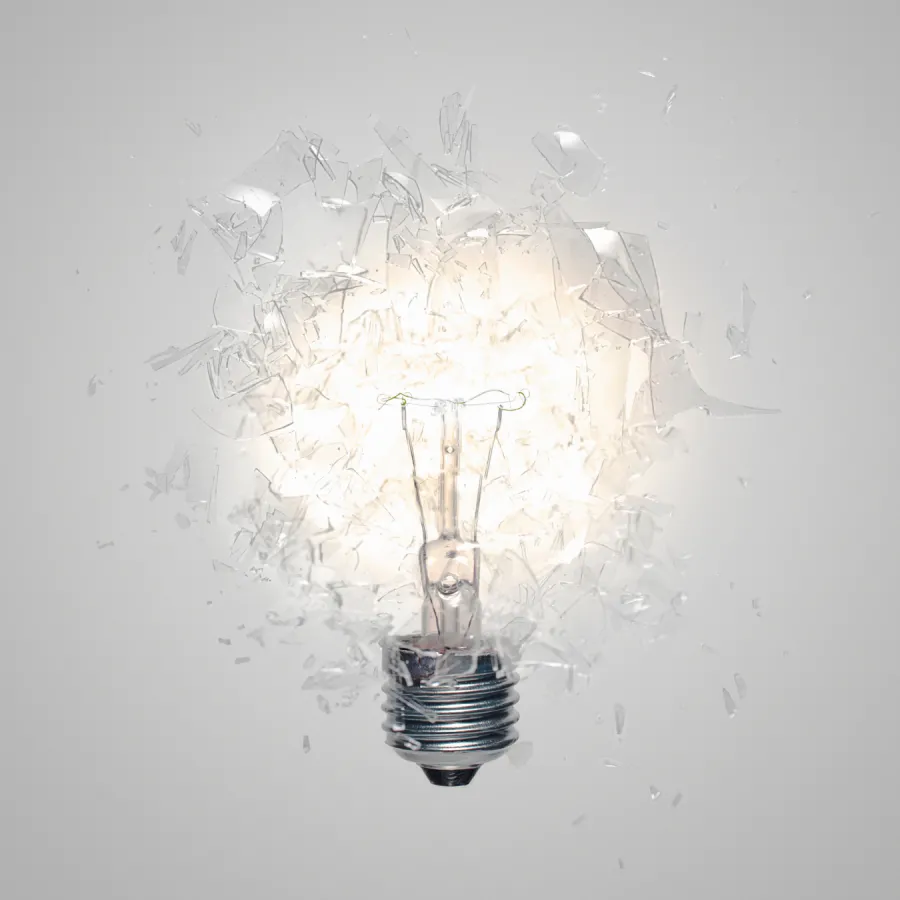
Nuix Notes: Monthly updates from the CEO - Jul24
Read more ›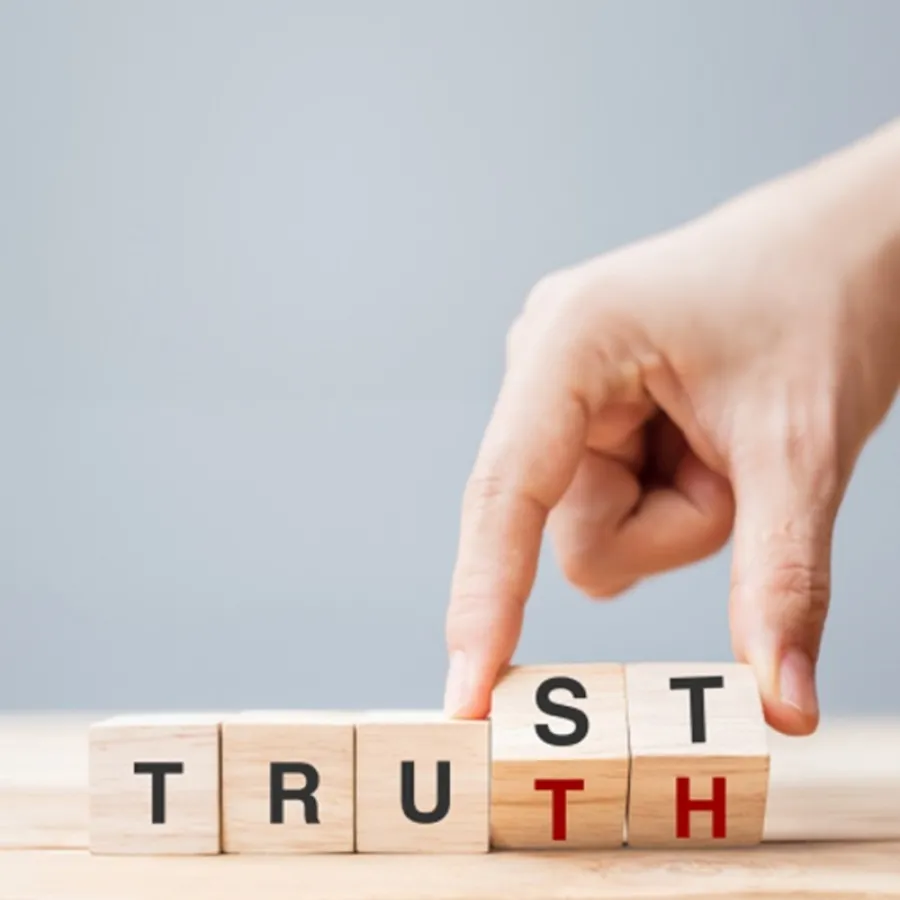
Xplore_AI: A Matter of Trust
Read more ›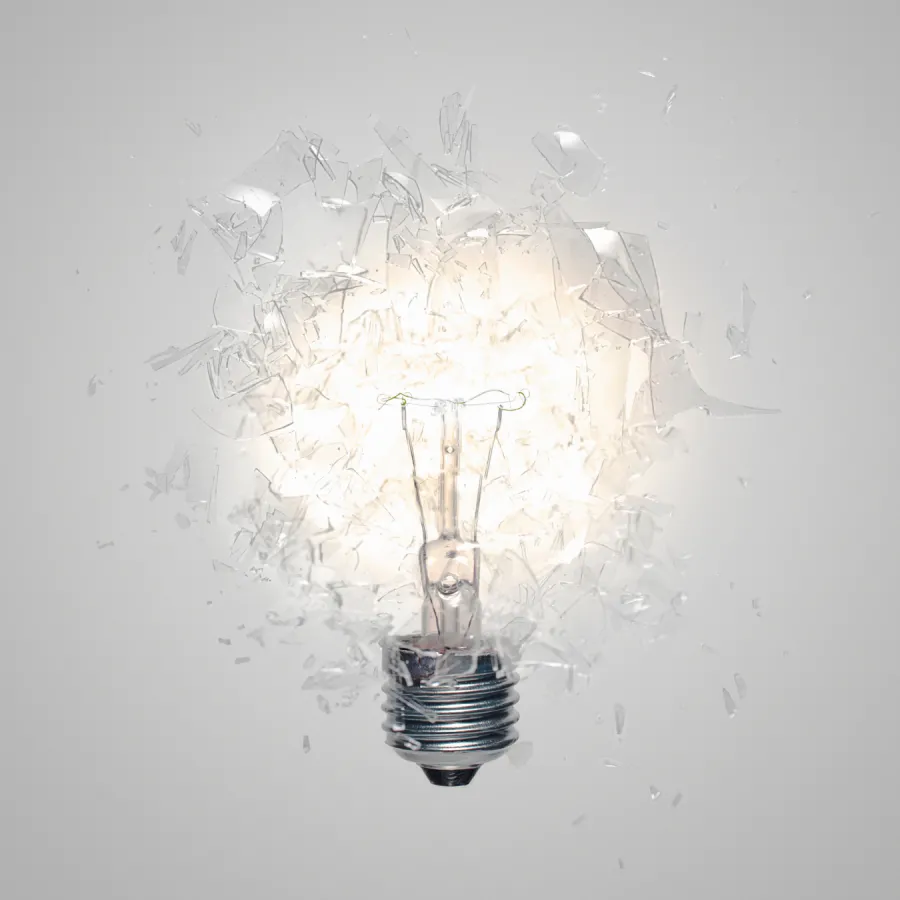
Nuix Notes: Monthly updates from the CEO - Jun24
Read more ›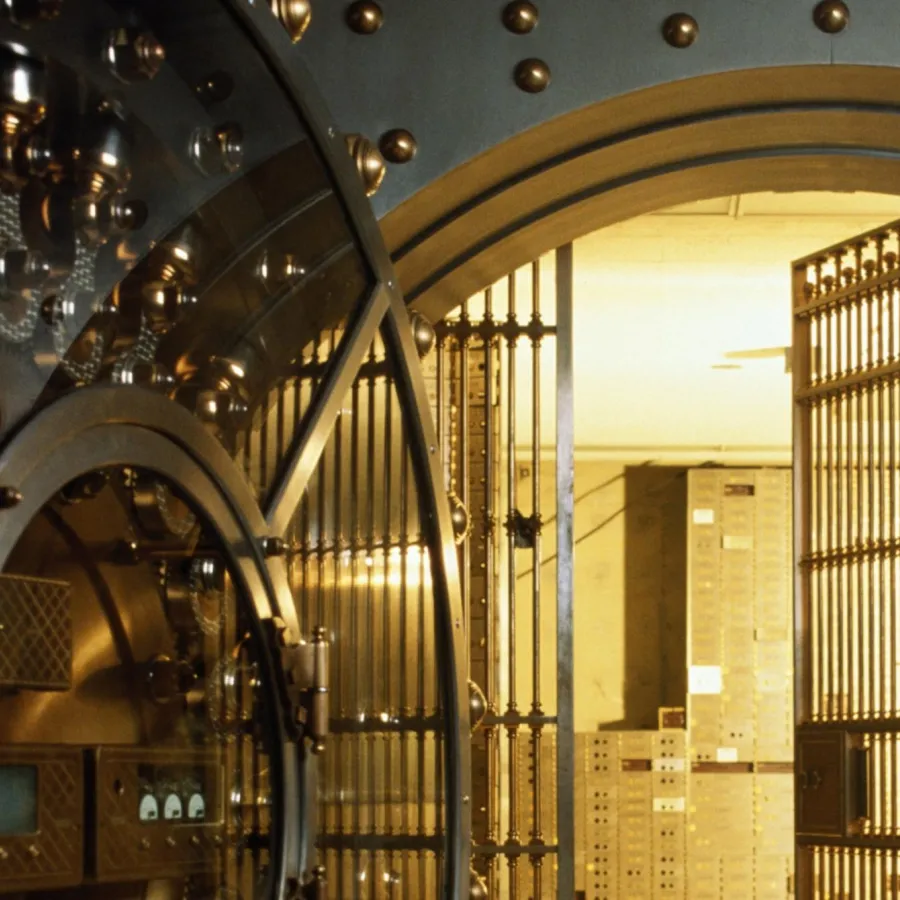
Xplore_AI: Into the Breach!
Read more ›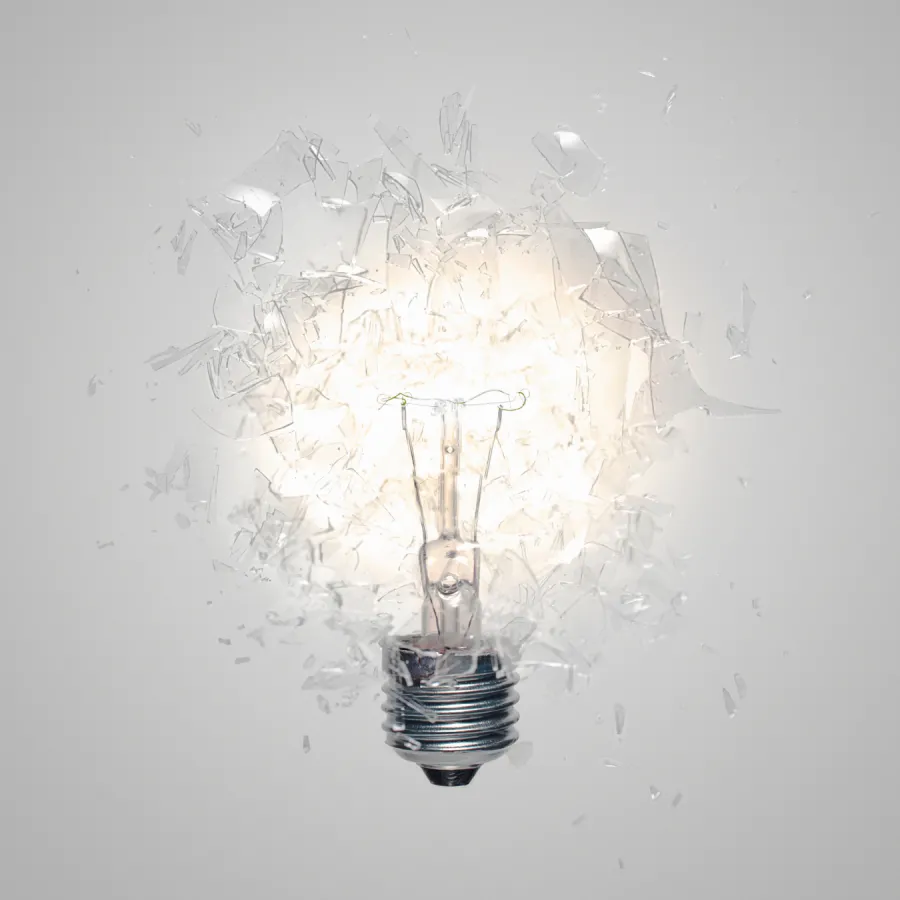
Nuix Notes: Monthly updates from the CEO - May24
Read more ›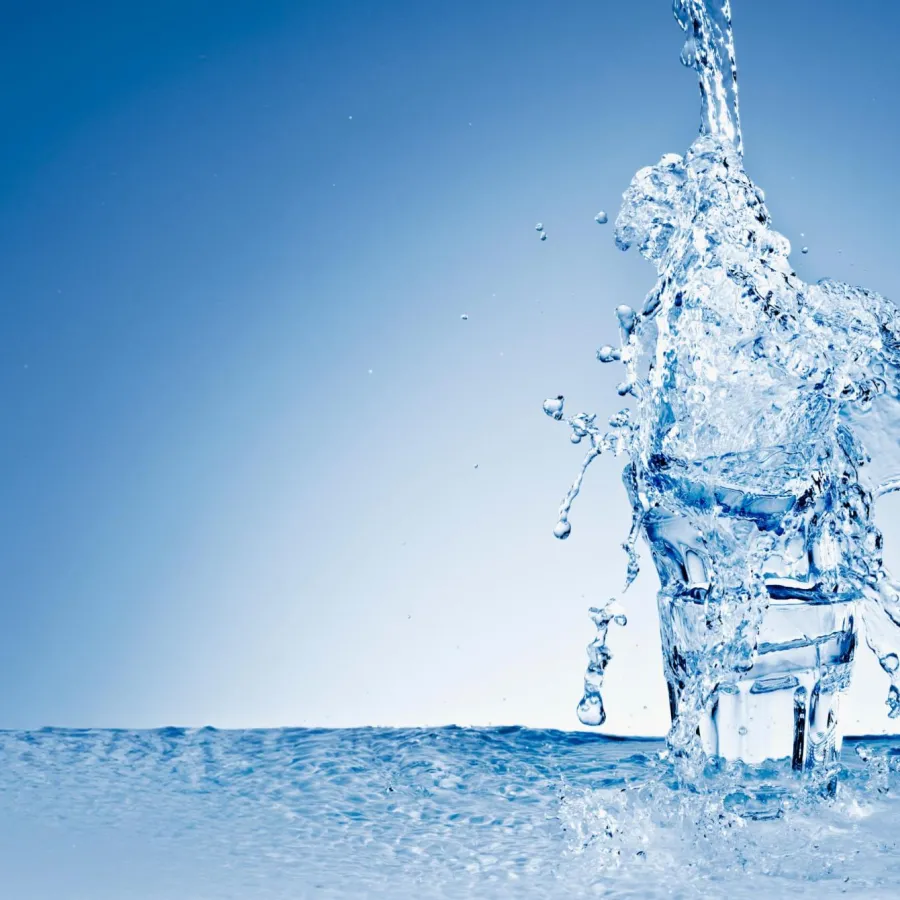
Xplore_AI: Water, water everywhere
Read more ›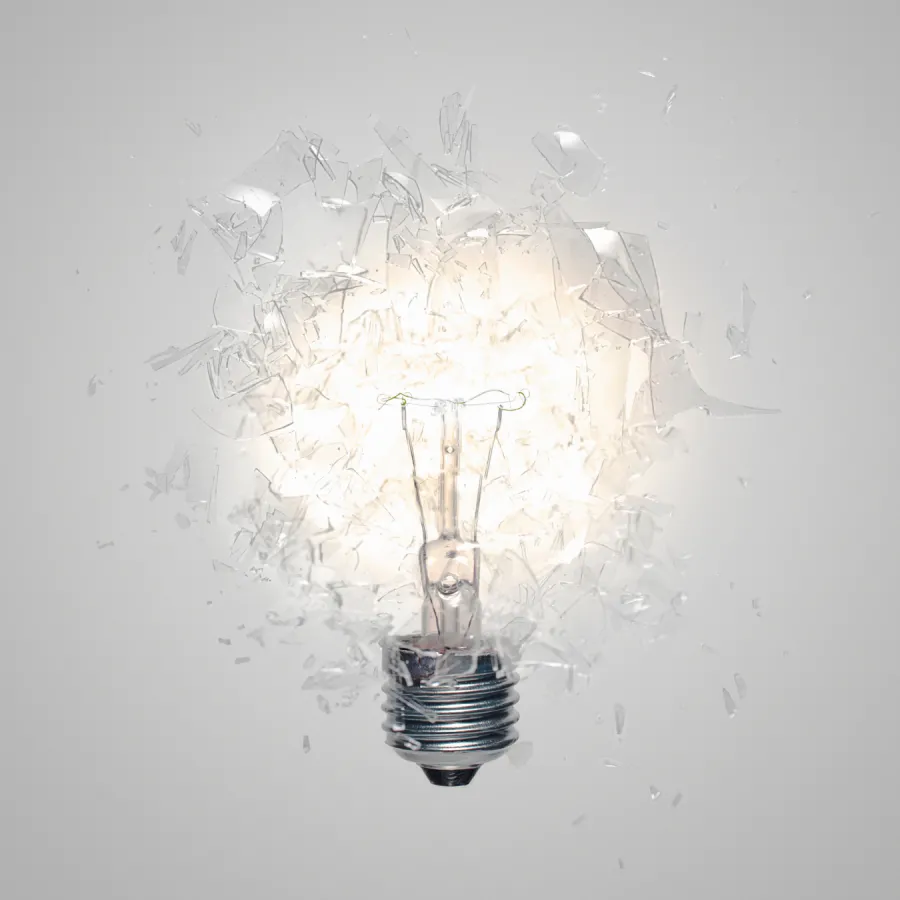
Nuix Notes: Monthly updates from the CEO - Feb24
Read more ›
Fact Sheets
Fact Sheets
White Papers
White Papers
Videos
Videos
Use Case
Use Case
Brochures
Brochures
Podcasts
Podcasts
Frequently asked questions
Our fiscal year runs from 1 July to 30 June.
Our ASX code is NXL.
Our auditors are KPMG.
A copy of the Annual Report can be downloaded from the Annual Reports section in the Investor Centre